Researchers have harnessed the power of artificial intelligence to significantly advance the discovery and optimization of multicomponent metal oxide electrocatalysts for the oxygen reduction reaction (ORR).
This breakthrough has the potential to revolutionize the efficiency and affordability of renewable energy technologies such as hydrogen fuel cells and batteries, paving the way for a sustainable energy future.
Details of the findings were published in the Journal of Materials Chemistry A on April 23, 2024.
The study analyzed 7,798 distinct metal oxide ORR catalysts from high-throughput experiments. These catalysts, containing elements such as nickel, iron, manganese, magnesium, calcium, lanthanum, yttrium, and indium, were tested at different potentials to evaluate their performance. Using the XGBoost machine learning method, the researchers built a predictive model to identify potential new compositions without the need for exhaustive experimental testing.
The research found that a high number of itinerant electrons and high configuration entropy are critical features for achieving high current density in ORR. For current density at 0.8 VRHE, the ternary systems Mn-Ca-La, Mn-Ca-Y, and Mn-Mg-Ca showed significant potential for hydrogen fuel cell applications. At 0.63 VRHE, the Mn-Fe-X (X = Ni, La, Ca, Y) and Mn-Ni-X (X = Ca, Mg, La, Y) systems were identified as promising candidates for hydrogen peroxide production.
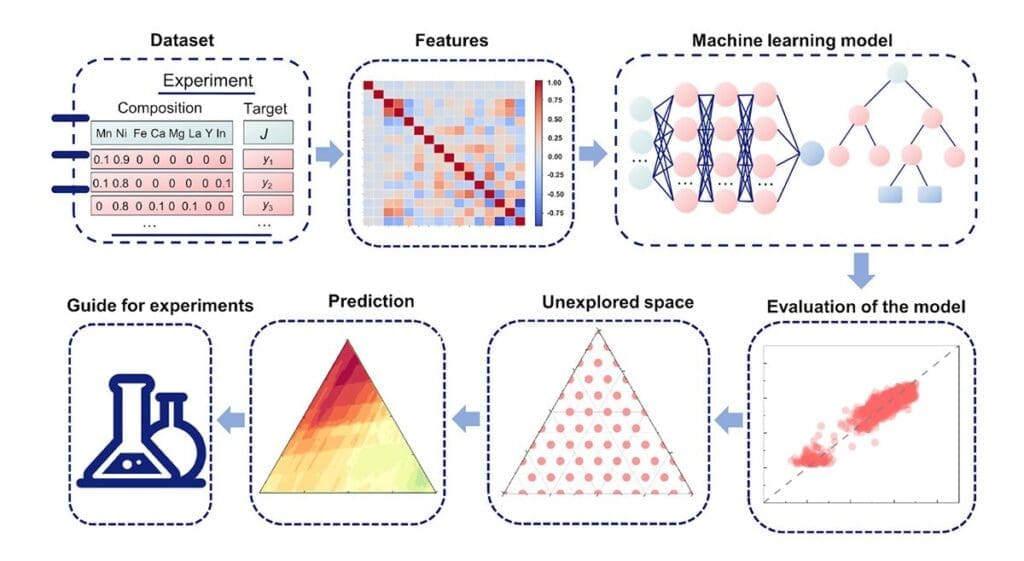
“Our innovative approach using machine learning accelerates the design and optimization of multicomponent catalysts, saving considerable time and resources,” says Xue Jia, Assistant Professor at the Advanced Institute for Materials Research and co-authors of the study. “By identifying high-performance catalyst compositions efficiently, we have a demonstrated transformative method that can lead to significant advancements in sustainable energy technologies.”
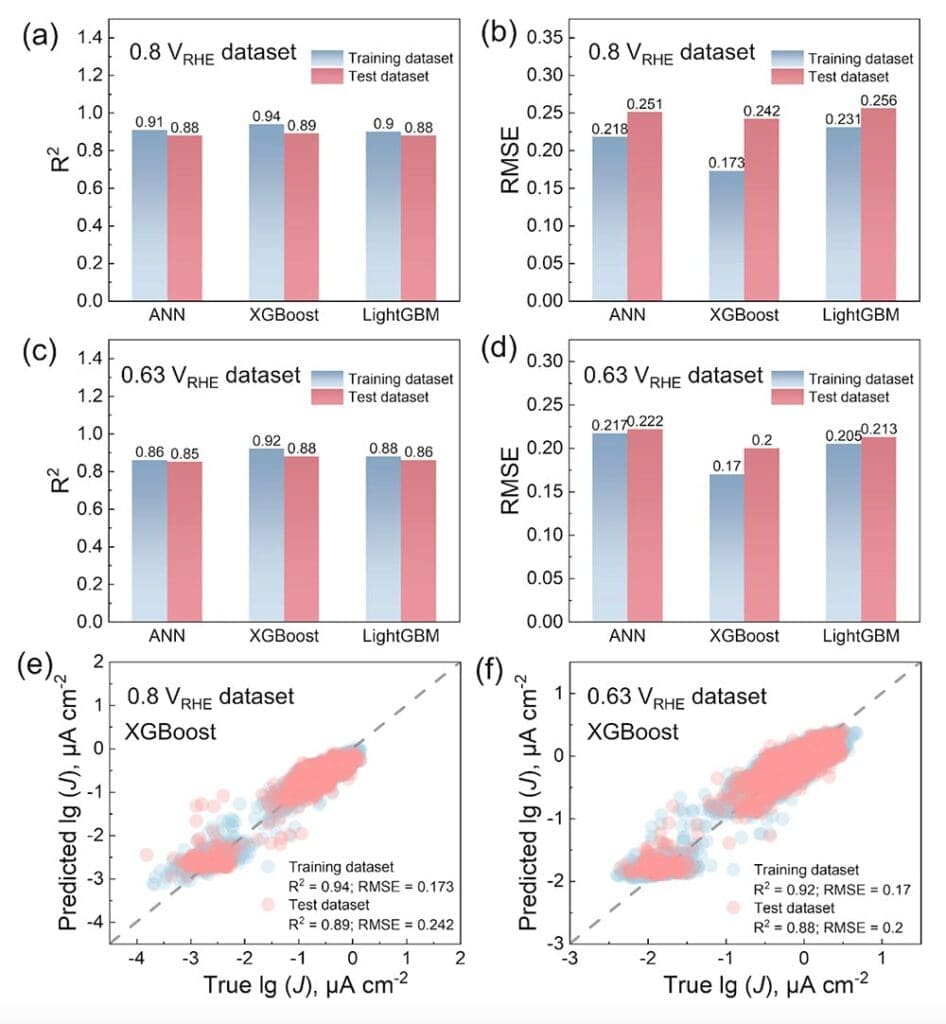
Enhanced catalysts can improve the efficiency and reduce the cost of renewable energy technologies, promoting their broader adoption and reducing reliance on fossil fuels. More efficient energy storage systems can lower overall costs, making renewable energy more accessible and contributing to environmental conservation.
The successful application of machine learning in this study sets a precedent for future research, potentially leading to breakthroughs in various scientific fields. Improved ORR catalysts can also enhance the production of hydrogen peroxide, widely used for disinfection and industrial processes, benefiting public health and safety.
“This research underscores the incredible potential of artificial intelligence in accelerating catalyst design and materials discovery,” adds Jia “Our findings will hopefully make future breakthroughs in sustainable energy technologies possible, which are crucial for addressing global energy challenges.”
Journal Reference:
Xue Jia, Hao Li, ‘Machine learning enabled exploration of multicomponent metal oxides for catalyzing oxygen reduction in alkaline media’, Journal of Materials Chemistry A 12, 12487-12500 (2024), DOI: 10.1039/D4TA01884B
Article Source:
Press Release/Material by Tohoku University
Featured image credit: vecstock | Freepik